AI Foundation Models in Biotech: New Paradigm
The rise of AI foundation models in biotech, companies on the cutting-edge. Biology is a new frontier. Data is still a bottleneck.
Hi! I am Andrii Buvailo, and this is my weekly newsletter, ‘Where Tech Meets Bio,’ where I talk about technologies, breakthroughs, and great companies moving the industry forward.
If you've received it, then you either subscribed or someone forwarded it to you. If the latter is the case, subscribe by pressing this button:
Now, let’s get to this week’s topics!
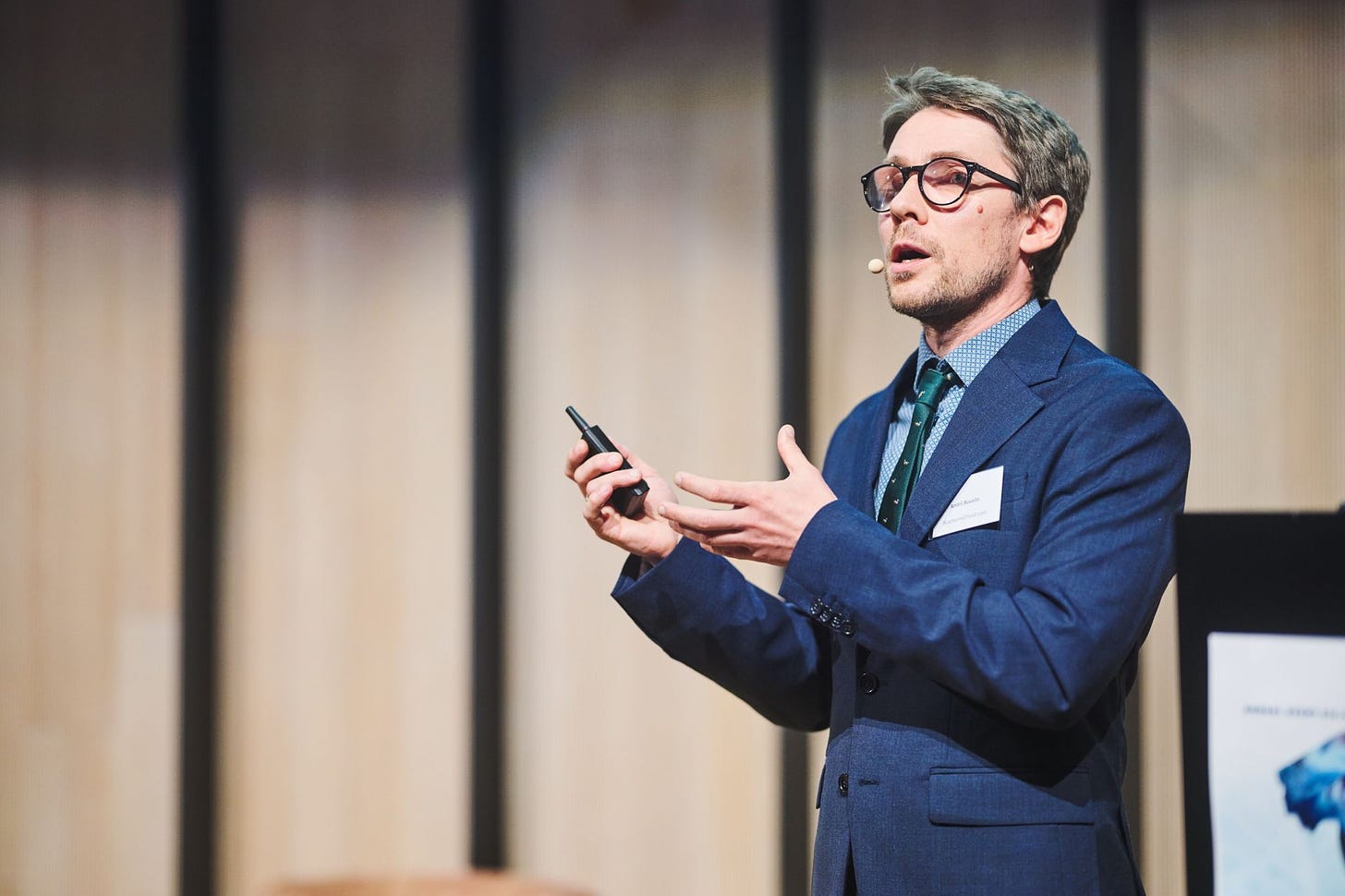
The rise of foundation models in life sciences was one of the central topics of my keynote speech this week during an annual industry event in Brussels, Belgium, organized by Bio.be (part of essenscia). Essenscia is the Belgian federation representing biotech and the life sciences industry. In Belgium, they seem to take artificial intelligence adoption in life sciences very seriously, since even Alexander De Croo, Prime Minister of Belgium, was speaking at the event.
But what are AI foundation models anyway, and why is all the buzz lately? And most importantly, why should we care about this in the life sciences?
A historical note
As you know, deep learning models have been trending ever since 2012, after a famous ImageNet competition where a deep convolutional neural network, AlexNet, achieved outstanding performance for image classification tasks, marking the beginning of the deep learning revolution in computer vision, with convolutional neural nets (CNNs) playing a key role.
While CNNs are great for grid-like data structures, such as images and video frames, they are not good for sequential data, like time series. So, recurrent neural nets (RNNs) and LSTMs have joined the leaderboards of deep learning popularity quite soon, in particular in biology research.
CNNs, RNNs, and other deep learning architectures were quite popular in drug discovery, with lots of startups being founded during 2013–2017 exploiting the idea of AI-driven drug design. Some of these companies are now unicorns with AI-inspired molecules already in clinical development—Exscientia, Insilico Medicine, BenevolentAI, and Relay Tx, to name a few.
Notably, machine learning strategies of those years were primarily focused on supervised learning based on a properly laballed dataset, and datasets were relatively small (up to millions or hundreds of millions of parameters at best). Most importantly, the AI models of this period are specific to a certain narrow task. For instance, modeling target-ligand interaction or de-novo generation of small molecules biased towards desired properties, etc.
A really remarkable development occurred in 2017, a pivotal year for the AI field. Google engineers introduced a so-called transformer architecture for neural nets and a mathematical algorithm of attention in a seminal paper, ‘Attention is all you need.‘
This changed completely the playing field of deep learning and marked a paradigm-shifting rise of larger and more generalizable models. The most famous series of models includes the GPT-X family, with GPT-3 and GPT-4 being the foundations of ChatGPT, a recent phenomenon that I am sure you know well enough without my explanations.
What are foundation models?
A foundation model represents an AI neural network extensively trained on vast amounts of unprocessed data through unsupervised (self-supervised) learning, enabling its adaptability to a wide array of tasks.
Two key concepts underpin this overarching category: the simplification of data collection and the expansive range of opportunities it presents. Foundation models primarily learn from unlabeled datasets, eliminating the need for manual data labeling.
In contrast to earlier neural networks, which were narrowly specialized for specific tasks, foundation models can easily transition to various roles with minor adjustments, from text translation to medical image analysis.
Finally, foundation models can be fine-tuned towards domain-specific tasks, like chemistry, biology, mathematics, and so on. This is done by additional training of a foundation model, pre-trained on non-domain-specific data, on a domain-specific dataset.
Now, a crucial aspect of what makes foundation models so unique and different from previous eras of AI is the concept of ‘emergent abilities’. With the increasing number of parameters for training, the models start spontaneously acquiring novel and expanding capacities in various areas—pretty much like a child who first learns many different patterns and then suddenly gets visibly better at everything at once.
Understandably, emergent abilities may give rise to unexpected and very valuable properties of foundation models trained on biology data, which is already being proved experimentally by such companies as Recursion Pharmaceuticals.
The great filter
With all the paradigm-shifting promise of foundation models in drug discovery and biotech, it seems like this technology is further increasing the market entry barrier for new life science startups. In order to achieve technological advantage, you may need to build foundation models for your R&D task, but in order to build a domain-specific foundation model, you need a lot of domain-specific data in a certain area. This is a real challenge for the overwhelming majority of companies, except for several of those possessing unique experimental data generation capabilities, such as high-throughput experimentation facilities.
As Chris Gibson, co-founder and CEO at Recursion Pharmaceuticals, writes in his LinkedIn post:
‘To build broad foundational models in biology, you are going to need a lot of high-quality data. Aside from a few problems (e.g., protein folding), that data doesn't currently exist in the public domain. The winners of TechBio will have access to high-quality talent, deep compute resources, AND the ability to iteratively generate rich biological data at scale...
One day we will move wet-lab biology to confirmation and validation of in silico hypotheses only, but only those who can generate data at scale and quality today will get to that point for most drug discovery and development problems...’
There are several companies that possess unique data generation abilities via in-house resources. Let’s review how they are building foundation models and what they are planning to do with them.