An Unnoticed Event That May Symbolize the Future of Drug Testing
ALSO: The new interface of Gen AI for drug discovery; weekly highlights; my favorite pharma industry yearly report; 5 foundation models in bio
Hi! I am Andrii Buvailo, and this is my weekly newsletter, ‘Where Tech Meets Bio,’ where I talk about technologies, breakthroughs, and great companies moving the biopharma industry forward.
If you've received it, then you either subscribed or someone forwarded it to you. If the latter is the case, subscribe by pressing this button:
P.S. I’ve had a crazy couple of days recently, so I had to delay last week’s update. But here we are, with even more insights.
Now, let’s get to this week’s topics!
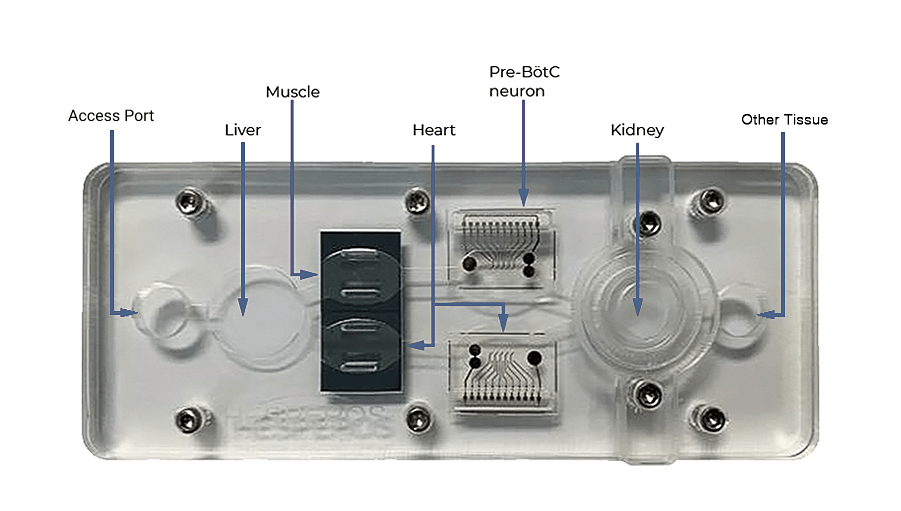
Today I'd like to highlight a quite interesting (a symbolic even?) event that went relatively unnoticed by media and science commentators. Did you know that in 2020, the US FDA greenlighted the first drug candidate to enter clinical trials based solely on efficacy data derived from study in organoids, without the use of animal models?
I didn't.
(Btw, thank you to Katya Tsaioun for sharing this article in her post, which popped up in my LinkedIn feed and led me to the idea of writing about this topic.)
The drug candidate in question is Sanofi SA’s sutimlimab (SAR-445088; formerly BIVV-020), a humanized anti-C1s monoclonal antibody. In the study NCT04658472 I am discussing today, this antibody is tested for a new indication: chronic inflammatory demyelinating polyneuropathy (CIDP). So, it is a drug-repurposing story.
The U.S. FDA OK'd the product under the brand name Enjaymo in February 2022 for the treatment of cold agglutin disease [CAD], in which activation of the complement cascade leads the immune system to destroy healthy red blood cells.
In the study I am highlighting today, Sanofi took that [CAD] safety data, combined it with efficacy data solely from a microphysiological system-based study for CIDP, and that was the only data that was presented to the FDA for a new indication.
“That’s the first time this has happened” according to James Hickman, CEO of Hesperos Inc. a company that developed the microphysiological system (human-on-a-chip) used in the study.
The study started recruiting just over two years ago, but the details were shared publicly only later, in 2022, following the publication of a peer-reviewed paper titled Classical Complement Pathway Inhibition in a ‘Human-on-a-Chip’ Model of Autoimmune Demyelinating Neuropathies. The paper describes the use of a human-on-a-chip system to mimic disease mechanisms of rare autoimmune neuropathies that cannot be replicated in animal models.
This development offers a glimpse into the potential future of drug testing without traditional animal models (aka in vivo), especially for diseases that can't be adequately simulated in animal models.
Why care?
In fact, there are over 10,000 rare diseases worldwide (there are varying numbers of rare diseases depending on the source), but only about 400 are being actively researched due to a lack of animal models.
Chronic inflammatory demyelinating polyneuropathy (CIDP) and multifocal motor neuropathy are rare, autoimmune neuropathies characterized by muscle weakness commonly resulting in difficulty ambulating and impaired hand function. Both are characterized by immune system hyperactivity through autoantibody production, leading to peripheral nerve demyelination and a reduction in nerve conduction velocity. While treatment with IVIG and steroids is available to patients, there is still a significant unmet need for novel, targeted treatments, which are lacking due in part to the absence of ideal animal models and of robust preclinical models that accurately mimic human disease.
Hesperos's organoid model revealed that a murine antibody could restore neuron function impaired by CIDP. Consequently, Sanofi SA’s sutimlimab was repurposed for CIDP treatment, combining prior safety data with the new efficacy findings. Remarkably, these combined data alone drove the FDA's approval decision, a solid milestone for the field of organ-on-a-chip systems.
Beyond CIDP, Hesperos is enthusiastic about leveraging microphysiological systems for other rare diseases. The long-term vision is to build enough confidence in these models for them to be accepted in studying more prevalent diseases.
The Broader Context
Biomedical Revolution: Thomas Hartung from Johns Hopkins University sees this development as a part of a larger revolution in bioengineering human organs. He highlighted how organoids were instrumental in understanding how the SARS-CoV-2 virus affected the human brain, a study that couldn't be conducted in animal models.
Addressing the Reproducibility Crisis: The emerging dominance of microphysiological systems indicates a potential solution to the "reproducibility crisis" in biomedical research. Traditional cell culture methods are laden with inconsistencies and inaccuracies. Microphysiological systems provide a more standardized and accurate approach.
Moving Towards Standardization: Emphasizing the rapid progress in this field, the Good Cell and Tissue Culture Practice (GCCP) guidance underwent a revision in 2022, with the new GCCP 2.0 aiming to address the advancements and intricacies of cell models and to uphold the highest quality standards.
The embracing of organoids and MPS by regulators and the biomedical community heralds a transformative era in drug research and testing. While this is just the beginning, the potential implications for treating rare diseases and enhancing research quality are substantial. As well as the business and investment potential of this complex and multifaceted sector.
FDA Modernization Act 2.0
I've been covering the area of organ-on-a-chip systems (and other emerging alternatives to animal testing) for quite some time now. Certainly, an important factor that is now playing in favor of many emerging companies in this space is a recent FDA Modernization Act 2.0 that became law on December 29, 2022.
Previously, it was a legal requirement for all drugs to be tested in animal models before first-in-man trials. The FDA Modernization Act 2.0 has expanded the range of nonclinical models that can be used to progress drugs to clinical studies (including organ-on-a-chip systems, cell-based assays, computer modeling, and some other systems), but also recognizes that animal models are still needed in some cases.
Genialis (approved image), biomarkers in clinical trials, also suggest recent newsletter on the same topic with lantern
Pick of the Week
Recently, I visited Pharma.AI Day by Insilico Medicine, a Hong Kong/New York-based pioneer of generative AI for drug discovery.
Pharma.AI is an end-to-end drug discovery platform that includes three key modules: PandaOmics for building hypotheses and target discovery; Chemistry42 for generative drug design; and Medicine42 for predicting success and optimizing clinical trials.
This platform is now used by over 40 leading pharma companies and has produced an internal pipeline of 31 therapeutic assets across 29 targets, including cancer, fibrosis, and central nervous system diseases, with four in clinical stages. The company’s lead drug, designed to treat idiopathic pulmonary fibrosis, is the first AI-designed drug for an AI-discovered target to reach Phase II trials with patients. Another drug developed by this platform—a potentially best-in-class USP1 cancer inhibitor for BRCA-mutated tumors—was recently licensed to Exelixis for $80 million upfront and additional milestone and royalty payments.
The new features of their generative AI platform had a "wow" effect on me. But first things first.
There was a “meaty” presentation by Alex Zhavoronkov founder and CEO of Insilico, and I just printscreened a couple of points from his slides that I guess are worth mentioning:
For instance, with Insilico’s Pharma.AI, it is possible to do this:
Alex also pointed out that in the AI era, those who can produce proprietary biological and chemical data at scale will gain a strategic advantage. Insilico has built a fully automated robotic laboratory for biology research and drug design in the city of Suzhou, China.
The lab features many things, including sample registration, cell cultures, compound management, HTS, high-content imaging, NGS, etc.
Apparently, they care about the future of their AI business model.
Now, some of the new features added to the Pharma.AI suite are:
Randomly, what I remember:
There is now an indication prioritization tool. Also, there is a new feature of automated gene-disease reports where you can crawl and summarize the association data with Gen AI.
In Chemistry42 there is now a new interactive way to set up experiments in the laboratory. Also, there are now 26 new models for ADMET predictions (generation, optimization, and annotation). There are new UI components to create new reward functions.
There is a new Alchemistry system, it calculates free binding energy (BE) for the generated molecules and compares molecules by BE. In theory, you don’t need any additional physics tools.
Also, there is a new module for kinases:
and lots of updates to the inClinico software:
But the one feature that is especially cool is Copilot, which is essentially ChatGPT for drug discovery.
The Copilot is connected to Pharma.AI platform and is fine-tuned on Insilico’s data. What it means is that you can basically talk to the platform and search for new ideas that you can then explore further. With this, you do not even need to know how it works. You just need to be a good biologist and know what to ask and where to move the conversation. The system has all the data and lots of potential answers.
I am a big fan of generative AI, and I am using dozens of tools myself. We also integrated custom GPTs into our BiopharmaTrend.com market research platform. And I do believe this is the future of interaction with digital resources and databases.
So, I liked this new feature a lot. It is nice when you can have a meaningful conversation with your lab software!
Weekly Highlights
Genentech and NVIDIA's collaboration integrates Genentech's AI capabilities and vast biological data with NVIDIA's accelerated computing and AI, specifically enhancing Genentech's AI for drug discovery. Key technical elements include the optimization of Genentech's proprietary machine learning algorithms on NVIDIA's DGX Cloud, a platform featuring dedicated AI supercomputing and software like NVIDIA BioNemo for generative AI in drug discovery.
The partnership also emphasizes a synergistic "lab in a loop" approach, where experimental data continuously informs and refines computational models. Genentech will have to be careful when sharing data with the external generative models, as the company pointed out.
Chroma's innovation lies in its ability to program specific protein properties, revolutionizing protein design in pharmaceutical applications. The AI demonstrated at least a 3% success rate in producing proteins with desired characteristics, showing promise in engineering proteins for complex diseases.
This breakthrough could pivot the focus from just creating feasible protein structures to designing proteins based on intended functions, potentially addressing currently untreatable diseases by targeting complex or challenging protein interactions.
This platform, positioned with exclusive rights to a vast physical and cloud-based data lake, contains over 40 years of de-identified patient health records and biospecimen data. This repository aids biopharmaceutical companies by providing insights for diagnostic and therapeutic development.
This partnership integrates Neo4j's knowledge graphs and native vector search with AWS's Amazon Bedrock, aiming to reduce generative AI hallucinations and improve the accuracy, transparency, and explainability of AI results. This collaboration addresses the need for long-term memory in large language models (LLMs) that are tailored to specific enterprise data and domains. Neo4j's capabilities in creating knowledge graphs and vector search are key to grounding LLMs for more reliable AI systems.
Digging Gems
Atlas Venture 2023 Year in Review by Bruce Booth is a must-watch (YouTube). In my opinion, this is one of the best industry reviews — by far.
5 Case Studies of Using Foundation Models in Biotech: