The Growing Momentum for AI Foundation Models in Biotech
ALSO: A Breakthrough in 3D Protein Structure Prediction of Large, Complex Protein Structures
Hi! I am Andrii Buvailo, and this is my weekly newsletter, ‘Where Tech Meets Bio,’ where I talk about technologies, breakthroughs, and great companies moving the biopharma industry forward.
If you've received it, then you either subscribed or someone forwarded it to you. If the latter is the case, subscribe by pressing this button:
Now, let’s get to this week’s topics!
Weekly Tech+Bio News:
💰 Zephyr AI secures $111M in Series A funding to advance its AI-driven tools for oncology and cardiometabolic diseases, aiming to enhance data analysis speed, expand datasets, and grow its teams to promote the democratization of precision medicine.
💰 Tierra Biosciences raises $11M in Series A funding to accelerate its AI-guided platform for rapid custom protein synthesis, targeting pharmaceutical, industrial, and agricultural applications.
🚀 AION Labs launches CombinAble.AI, a new startup using AI for the rapid and cost-efficient design of targeted antibodies, aiming to significantly improve the drug development process by integrating AI with computational biomolecule simulations for more effective therapeutics.
🔬 Lantern Pharma initiates first-in-human clinical trial of AI-guided drug-candidate LP-284 for treating relapsed or refractory non-Hodgkin’s lymphoma and solid tumors, highlighting the potential to improve outcomes for 40,000 to 80,000 blood cancer patients annually with a market potential of $4 billion USD.
A Breakthrough in 3D Protein Structure Prediction of Large, Complex Protein Structures
Basecamp Research announced the development of BaseFold, a new deep learning model designed to predict the 3D structures of large, complex proteins with unprecedented accuracy.
This model represents a significant advancement over existing AI-powered tools, including the widely recognized AlphaFold2. The introduction of BaseFold is expected to accelerate the pace of AI-based drug discovery by offering more reliable predictions for the structures of larger and more complex proteins.
BaseFold's enhanced predictive capabilities stem from its use of BaseGraph, a comprehensive foundational dataset built by Basecamp Research.
BaseGraph has been assembled through partnerships with over 25 biodiversity-rich countries, aiming to capture a vast array of genetic information far beyond what current public protein databases offer.
These databases, often criticized for their limited size and scope, are believed to represent a minuscule fraction of life on Earth, restricting the effectiveness of AI tools in predicting protein structures that are not well-represented in these datasets.
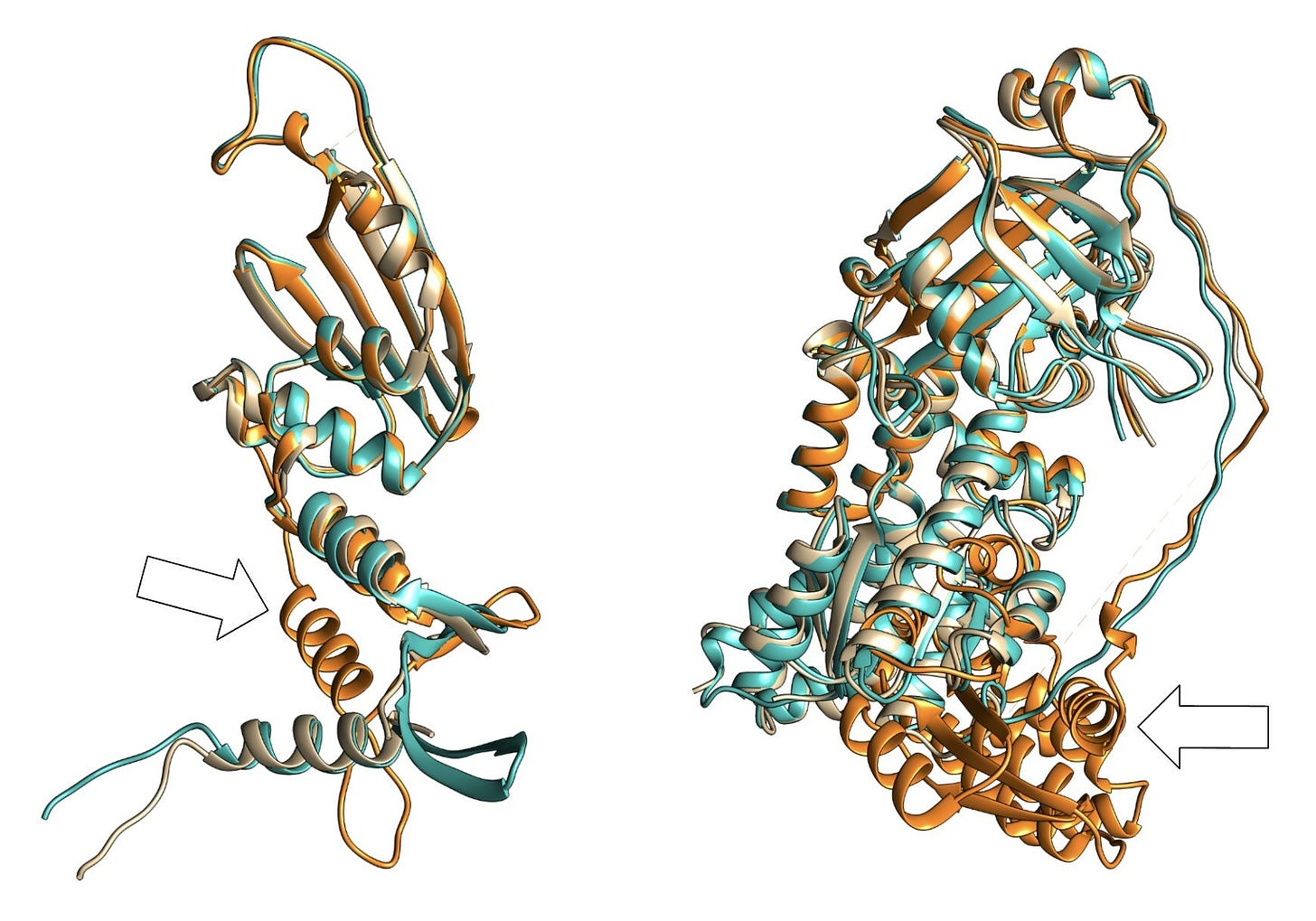
By integrating over 6 billion relationships contained in BaseGraph, BaseFold can extract significantly more evolutionary information, enabling it to predict protein structures and small molecule interactions with much greater accuracy.
The company published results claiming a sixfold improvement in accuracy over AlphaFold2 for certain proteins and up to threefold better accuracy in modeling small molecule interactions with protein targets.
The limitations of current AI models, including AlphaFold2, are partly due to their reliance on public databases like MGnify, which suffers from issues such as incomplete sequences. These issues can degrade the quality of predicted structures, especially for larger proteins. BaseFold aims to overcome these challenges by achieving an accuracy comparable to traditional, time-consuming experimental methods like X-ray crystallography, especially for proteins underrepresented in existing databases.
Basecamp Research's collaboration with NVIDIA to optimize BaseFold for the NVIDIA BioNeMo platform underscores the ongoing efforts to make this tool more accessible and effective for drug discovery.
Dr. Philipp Lorenz, CTO of Basecamp Research, emphasized the importance of diverse, representative genomic data for advancing AI in biotechnology. The team's effort to collect and annotate biodiversity data with precision marks a significant step forward in building datasets that are purpose-built for the AI era.
Meanwhile, Dr. Glen Gowers, co-founder of Basecamp Research, highlighted the limitations of current AI tools in predicting the structure of large, complex proteins and underscored the critical role of high-quality data in producing accurate AI outcomes.
The Growing Momentum for AI Foundation Models in Biotech and 12 Notable Companies
As artificial intelligence (AI) foundation models grow increasingly capable, they become useful for applications across a wide range of economic functions and industries, including biotech.
The most prominent examples of general purpose foundation models are the GPT-3 and GPT-4 models, which form the basis of ChatGPT, and BERT, or Bidirectional Encoder Representations from Transformers.
These are gigantic models trained on enormous volumes of data, often in a self-supervised or unsupervised manner (without the need for labeled data).
Thanks to special model design, including transformer architecture and attention algorithms, foundation models are inherently generalizable, allowing their adaptation to a diverse array of downstream tasks, unlike traditional AI models that excel in single tasks like, say, predicting molecule-target interaction.
The "foundation" aspect comes from their generalizability: once pre-trained, they can be fine-tuned with smaller, domain-specific datasets to excel in specific tasks, reducing the need for training new models from scratch. This approach enables them to serve as a versatile base for a multitude of applications, from natural language processing to bioinformatics, by adapting to the nuances of particular challenges through additional training.
Foundation models in bio
A number of companies are racing towards building more domain-specific foundation models, with even more accuracy and relevance than all-purpose models.