The Rise of SynBio in the Era of AI
Stages of AI-driven biologics design; looking at some cutting edge companies merging Synbio with AI -- from gene editing and multi-kilobase RNA editing, to pathway design
Hi! I am Andrii Buvailo, and this is my weekly newsletter, ‘Where Tech Meets Bio,’ where I talk about technologies, breakthroughs, and great companies moving the industry forward.
If you've received it, then you either subscribed or someone forwarded it to you. If the latter is the case, subscribe by pressing this button:
I took a little break from publishing last week but am now back with more ideas and insights. Also, we have moved our newsletter to a dedicated domain, www.techlifesci.com. We have been growing steadily, and I think it is the right time to have a domain that reflects the essence of what we are exploring here. I hope you like it.
Now, let’s get to this week’s topics!
We are living in a century of biology. This is my sincere belief.
In terms of global impact, progress in biotech and synthetic biology in particular will outshine anything else, even things like artificial intelligence, within a decade or two. Mainly because of the sheer breadth of ways synthetic biology influences our lives, sustainability, the environment, and the global economy.
Going forward, progress in biology research will inevitably change the very way human evolution can unfold. Yes, think about the genetic engineering of germline cells. Or in vivo gene therapies. Or stem cells. Or serious longevity interventions, adding decades to an average lifespan. There is also promise in such areas as brain computer interfaces and next-generation prosthetics. We are in the early days, of course, but ultimately, the human body will someday be augmented or modified in ways that will pretty much change who we are. Potentially. Who knows. Let’s leave such speculations to transhumanists and futurists.
A Bit of Context for SynBio Revolution
In the past several decades, we've seen huge changes in biology, especially in how we work with living systems. A major part of this change came from the "genomic revolution," where we learned how to read the genetic information (DNA) of a cell at scale and at a low cost.
Probably, the most publicized event of this revolution was The Human Genom Project, which resulted in a rough draft of the human genome. It primarily used Sanger sequencing, also known as capillary electrophoresis sequencing, so it was a long and expensive endeavor that started in the 1990s and was declared complete in 2003.
What dramatically changed the game of getting the most fundamental biological information about DNA and RNAs at scale was the next generation sequencing revolution. It started in the early 2000s, and, within just two decades, led to a price drop of sequencing whole genomes from $100.000.000 down to less than $1000, a progress largely attributed to a genomic sequencing market monopolist Illumina.
Here is an amazingly summarized history of DNA sequencing and NGS by Immy Mobley, if you want to familiarize yourself with key milestones.
Thanks to this genomic revolution, we now have tools like CRISPR that let us change DNA with great accuracy. Think of DNA as a recipe for building a living thing, and the actual outcome of this recipe (like how proteins are made) is called the phenotype. By studying the relationship between DNA changes and these outcomes, we gain valuable insights into how life works. What is much more important, we can use that information to our advantage.
Already now, thanks to synbio, we have insulin production without relying on pig pancreases, lab-made leather, jackets crafted from spider silk without involving any spiders, and even drugs that combat malaria and cancer. There are also meat-free burgers that mimic the taste of real meat, eco-friendly biofuels, beer with a hoppy taste minus actual hops, and recreations of scents from long-extinct flowers. Moreover, innovations include synthetic collagen for beauty treatments and methods to combat mosquitoes carrying dengue.
So, from a practical standpoint, Synthetic biology, often shortened to "synbio," focuses on customizing biological systems for specific tasks, like making cells produce a certain amount of biofuel or respond to external triggers. Synbio takes cues from engineering, using its predictable methods to manage the intricacies of biology. Key to this approach is using consistent genetic components and following a four-step process:
Design: Conceptualize a DNA sequence or cellular changes to meet a goal.
Build: Put the design into action, typically by creating a DNA segment and introducing it to a cell.
Test: Collect and analyze data to see if the new design behaves as expected, noting any unexpected results or side effects.
Learn: Use the data to refine and improve the process. AI can play a role here, helping to guide future designs and make the process more efficient.
At its core, synbio zeroes in on making changes at the DNA level to get cells to produce specific substances or act in predefined ways.
How AI Enables Synthetic Biology in the Context of Drug Discovery
Initially used for small molecules, AI is now shaping the discovery of biologics, including antibodies, protein-based treatments, and gene therapies. These treatments are notable for their precise targeting and wide-ranging use in medical research.
For example, at Absci, researchers have recently combined extensive biological data with advanced AI techniques to develop unique antibodies, ones that conventional methods might overlook. Through a method known as zero-shot drug discovery, they've been able to design antibodies tailored to specific targets, even without prior data on antibodies for those exact sites. What sets this work apart is that these aren't just theoretical designs; they’re taken from digital concepts to tangible results in the lab. The entire process, from initial design to laboratory validation, is completed in approximately six weeks.
Zero-Shot Learning models are developed primarily to extend a pre-trained model to novel classes when data from these become incrementally available— for example, adapting a classifier initially trained to distinguish between dogs and cats using supervised learning to classify birds on the fly — www.v7labs.com.
Next, as Sean McClain, Absci Founder and CEO explains in his opinion post on SynBioBeta, synbio and deep learning can be harnessed to pinpoint new drug targets and corresponding antibodies.
Reverse immunology, rooted in natural immune responses, starts by examining patients who exhibit outstanding immune reactions. By taking the RNA sequences of these patients' immune proteins, scientists at Absci can digitally construct human antibody sequences. These sequences are then produced in the laboratory. From there, folks at Absci employ comprehensive proteomic screening to identify their corresponding target antigens, a process known as "deorphaning." By the end of this procedure, they possess both the immune target and a human monoclonal antibody, providing a foundation for subsequent drug development.
Optimizing biologics, especially antibodies, involves refining them in three key aspects: effectiveness, developability, and safety. Concerning safety, scientists at Absci can utilize AI to enhance antibody candidates based on what's termed the Naturalness score. This score evaluates how similar an antibody is to a vast pool of natural immunoglobulin sequences we've gathered. Notably, the team at Absci found that a higher Naturalness score typically correlates with a reduced risk of causing unintended immune reactions. By emphasizing this score during drug development, they aim to proactively address potential challenges related to developability and immunogenicity, giving preference to the most "natural" antibody candidates.
The manner in which antibodies connect with target proteins in our bodies is crucial for their effectiveness. This includes how antibodies interact with one another and how minor sequence adjustments can enhance their binding strength. There are several AI tools that forecast protein interactions, utilizing ML techniques like Monte Carlo or the fast-Fourier transform to evaluate how energetically compatible two structures are when they bind. These methodologies can also assess antibody candidates for characteristics vital for developability, such as stability and solubility. Integrated into a comprehensive drug development system, these AI instruments streamline the selection of potential antibody candidates.
Amber Bio’s proprietary platform leverages multi-kilobase RNA editing and AI foundation models to expand the reach of treatable pathogenic variants
Current gene editing technologies have a significant limitation: they primarily address mutations individually, a method that's not scalable given the vast genetic diversity across patient populations globally. This has left many genetic disorders, caused by a myriad of mutations, largely untreatable.
However, Amber Bio, a biotech startup based in San Francisco, is striving to rewrite this narrative. With a recent infusion of $26 million in seed financing co-led by Playground Global and Andreessen Horowitz Bio + Health, the company is advancing its innovative RNA editing platform. Unique in its ability to conduct multi-kilobase edits, this platform could allow a single drug to treat diseases that stem from a broad array of mutations.
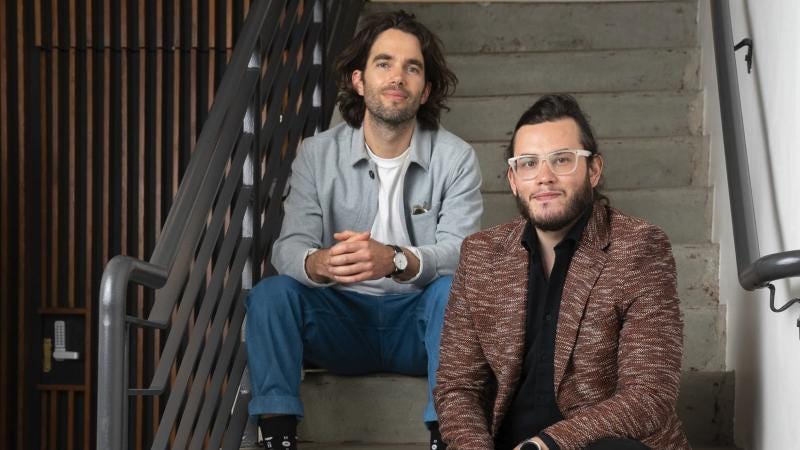
The company's mission, under the leadership of Co-founders Jacob Borrajo, Ph.D., CEO, and Basem Al-Shayeb, Ph.D., CTO, is to revolutionize RNA rewriting, providing a sustainable and more encompassing alternative to traditional DNA editing which may lead to irreversible mutations. This innovation, blending multi-kilobase RNA editing with AI foundational models, promises a new horizon for patients suffering from varied genetic disorders.
From Gene Editing to Pathway Design: How AI is Transforming Synthetic Biology
Considering the “data-rich” nature of biological experimentation and heavy reliance on such data, no wonder why artificial intelligence (AI) is increasingly playing a central role in advancing synthetic biology by facilitating the design, construction, and optimization of biological systems.
Let’s review six ways AI is used in various aspects of synbio, along with leading companies in each category