Weekly Tech+Bio Highlights #37: Turning Cells Into Language
Also: Industry Highlights, Who Runs the Tools in AI–Pharma Deals, and Four Challenges at the AI-Biology Interface
Hi! This is BiopharmaTrend’s weekly newsletter, Where Tech Meets Bio, where we explore technologies, breakthroughs, and cutting-edge companies.
Note: we’ve recently launched a new report outlining a framework for what defines modern AI-driven drug discovery, for 2025 and beyond.
If this newsletter is in your inbox, it’s because you subscribed or someone thought you might enjoy it. In either case, you can subscribe directly by clicking this button:
Let’s get to this week’s topics!
🤖 AI x Bio
(AI applications in drug discovery, biotech, and healthcare)
🔹 Open-source AI consortium OpenFold has added eight new members—Bristol Myers Squibb, COGNANO, Lambda, Novo Nordisk, Structure Therapeutics, Tamarind Bio, Unnatural Products, and Visterra—to expand collaborative development of free protein modeling tools for drug discovery in areas like cancer, metabolic disease, and immune disorders. This follows the addition of six new members in August 2024.
🔹 HealthVerity has partnered with Recursion to integrate real-world data from over 340M U.S. patients into Recursion’s AI-driven Recursion OS to enable smarter clinical trial design, patient recruitment, and in silico simulations.
🔹 Elix and PRISM BioLab have partnered to combine AI-driven compound design with scaffold chemistry to target hard-to-drug protein-protein interactions in diseases like cancer, fibrosis, and autoimmune conditions.
🔹 Researchers at Google and Yale have taught language models to interpret single-cell RNA data as text, enabling natural language analysis of cell states and drug responses; their open-source C2S-Scale models, up to 27B parameters, are trained on 1B+ tokens and available on HuggingFace and GitHub.
🔹 Cellino partners with Karis Bio to develop the first autologous iPSC therapy for cardiovascular disease, using AI-driven biomanufacturing to produce patient-specific cells for clinical use in artery repair.
🔹 Four research teams have been named finalists in the Coller Dolittle Challenge for using AI to study two-way communication with dolphins, nightingales, cuttlefish, and monkeys, competing for a $100K prize and a $10M Grand Prize for autonomous interspecies dialogue.
🔹 Researchers in Venezuela have developed a convolutional neural network to detect malaria in blood samples with 99.5% accuracy, using NVIDIA GPUs to support remote diagnosis amid a disease resurgence.
🔹 In an episode of NVIDIA’s AI Podcast, Isomorphic Labs’ Max Jaderberg and Sergei Yakneen describe their AI-first approach to drug discovery, framing biology as an information system and detailing efforts to simulate molecular interactions beyond traditional methods.
🔹 In a Nature perspective, Bo Wang and colleagues present a roadmap for multimodal foundation models (MFMs)—large AI models trained on multi-omics and time-series data—to serve as the computational backbone for building virtual cells and simulating context-specific gene regulation and in silico perturbations.
🔹 Kejun Ying at Harvard Medical School, announced MethylGPT, a transformer-based model trained on 226,555 methylation profiles from 5,281 datasets—reportedly the largest of its kind—to predict age, disease, and mortality across tissues; codebase available on GitHub.
🔹 Ono partners with Jorna Therapeutics to develop RNA editing drugs using Jorna’s generative AI platform, combining quantum mechanics-based modeling with large-scale sequence design to target previously inaccessible RNAs.
🔹 Charm Therapeutics CEO and founder Laksh Aithani steps down as the London-based AI biotech, which uses its co-folding platform to target hard-to-drug proteins, prepares for clinical entry in 2026.
🚜 Market Movers
(News from established pharma and tech giants)
🔹 Illumina partners with Tempus to expand DNA sequencing beyond cancer, aiming to train AI models on genomic data and integrate molecular profiling into routine care across diseases like cardiology, neurology, and immunology.
🔹 In The Wall Street Journal, Isabelle Bousquette reports that Johnson & Johnson has narrowed its generative AI strategy after a year of experimentation, with CIO Jim Swanson noting a shift from nearly 900 exploratory use cases to a prioritized focus on the 10–15% that drove around 80% of the value.
🔹 BigHat Biosciences has partnered with Eli Lilly to design AI-driven antibody therapeutics by combining BigHat’s ML-powered platform with Lilly’s drug discovery expertise, targeting two programs and advancing next-gen treatments for chronic diseases and GI cancers.
💰 Money Flows
(Funding rounds, IPOs, and M&A for startups and smaller companies)
🔹 GeneDx to acquire AI-driven genomic interpretation firm Fabric Genomics for up to $51M to enable decentralized genome analysis, combining GeneDx’s rare disease dataset with Fabric’s cloud-based platform for interpretation services.
🔹 Earendil Labs has licensed two AI-designed bispecific antibodies for autoimmune and inflammatory bowel diseases to Sanofi in a deal worth up to $1.72B, with a $125M upfront payment and near-term milestones.
🔹 RadNet acquires mammography AI developer iCad in a $103M all-stock deal to expand its DeepHealth platform, integrating iCad’s breast cancer detection tools and commercial team.
🔹 Austrian biotech a:head bio AG concluded a 7-digit funding round with existing and new investors to expand its human brain tissue platform, focusing on neurodegenerative disease models and platform automation for large-scale organoid production.
⚙️ Other Tech
(Innovations across quantum computing, BCIs, gene editing, and more)
🔹 Lab-grown teeth could replace fillings and implants as King’s College London and Imperial College researchers develop a cell-signaling material that mimics natural tooth formation, enabling cells to initiate early tooth development in the lab for future regenerative dental treatments.
🔹 The Wellcome Sanger Institute and PacBio have launched a long-read single-cell RNA sequencing initiative to decode immune gene activity in 1,500 blood and gut samples, aiming to map RNA isoform diversity linked to IBD and treatment response across diverse populations.
🔹 Two new studies in Nature have shown that transplanting lab-grown dopamine neurons from donor stem cells into Parkinson’s patients is safe and can ease symptoms, with improvements lasting up to two years—Shelly Fan, SingularityHub.
🏛️ Bioeconomy & Society
(News on centers, regulatory updates, and broader biotech ecosystem developments)
🔹 China could outspend the U.S. on R&D by over 30% by 2030, according to a Monte Carlo analysis by R&D World, with some scenarios forecasting a 60%+ gap—even amid trade tensions and CHIPS Act uncertainty—driven by sustained public investment and accelerated growth across China’s innovation economy.
🔹 Convergent Research has launched the Fundamental Development Gap Map, an online tool mapping key R&D bottlenecks in science alongside the mid-scale infrastructure—tools, technologies, and data—needed to address them, aiming to guide coordinated efforts and spark discussion across the research ecosystem.
🔹 Lisa Noble, diagnosed with B cell lymphoma, became cancer-free three months after receiving CAR-T cell therapy at Addenbrooke’s Hospital following unsuccessful chemotherapy. A new NHS-University of Cambridge lab, opening by 2026, will locally produce such therapies and develop new treatments for cancer and autoimmune diseases.
🔹 The Netherlands has released a national biotech strategy to position itself as a global leader by 2040, focusing on tools-first infrastructure, regulatory reform, and commercialization support to accelerate innovation in health, agriculture, and the circular economy.
🔹 The UK has launched a one-year rollout of new clinical trial regulations to cut approval timelines and red tape, aiming to reduce trial startup time from 250 to 150 days.
🚀 A New Kid on the Block
(Emerging startups with a focus on technology)
🔹 Seattle startup Potato has raised $4.5M to build AI assistants and lab robots to enable fully automated scientific experiments, aiming to cut costs, improve reproducibility, and accelerate discovery through a closed-loop research process.
The platform reportedly already supports labs at institutions like MIT, Stanford, and Harvard, using generative AI refined with scientific literature via RAG to assist with hypothesis generation, protocol design, and paper review, with plans to expand from life sciences into materials and chemistry.
This newsletter reaches over 8.4K industry professionals from leading organizations across the globe. Interested in sponsoring?
Contact us at info@biopharmatrend.com
Turning Cells Into Language
A team from Yale and Google Research (led by David van Dijk and Bryan Perozzi) introduced C2S-Scale, a new family of open-source large language models designed to work directly with single-cell RNA sequencing (scRNA-seq) data.
The core idea—represent each cell as a ranked list of its most expressed genes (a “cell sentence”) and use that as input for language models.
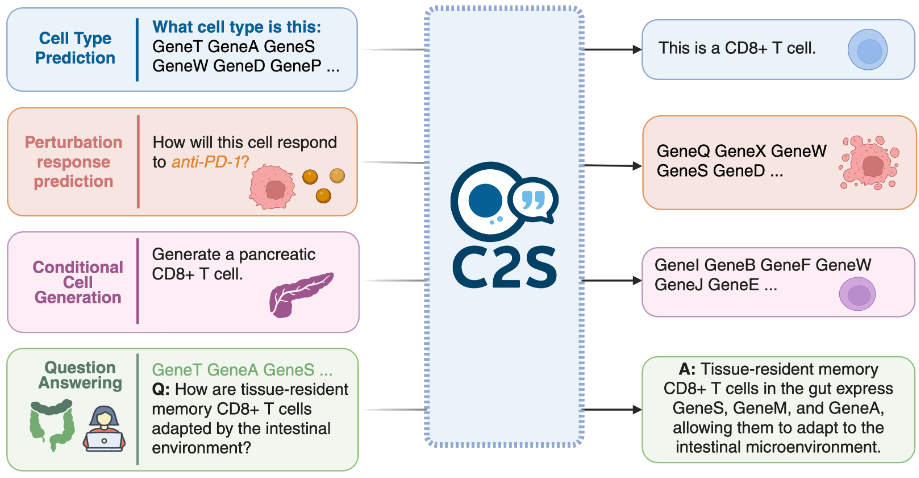
By converting high-dimensional expression vectors into text, these models can apply standard NLP tools to biological data. That includes asking questions like “What is this cell likely doing?” or simulating how it might respond to a drug or perturbation.
C2S-Scale models range from 410 million to 27 billion parameters. They’re based on Google’s Gemma architecture and trained on over 1 billion tokens from real transcriptomics data, biological metadata, and literature. The models are designed to cover a range of use cases, from smaller models for lower-compute environments to larger ones for more demanding biological reasoning.
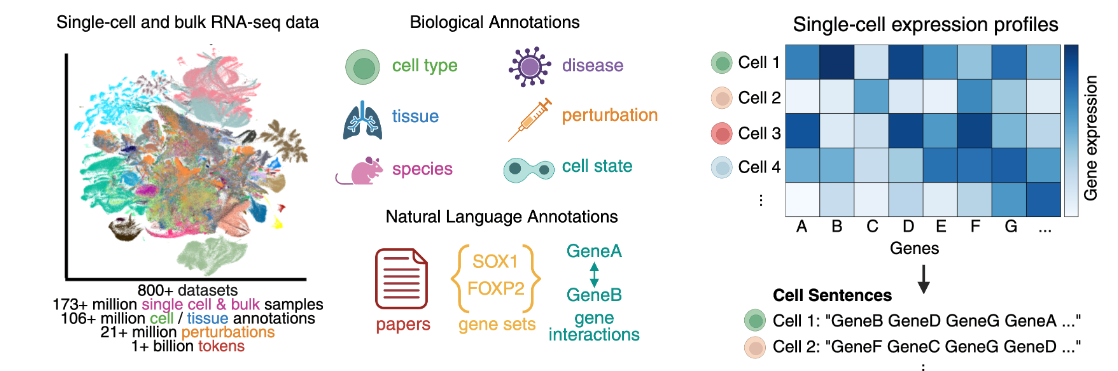
The models can handle both predictive and generative tasks. That includes generating descriptions of cell types or tissues, answering queries in natural language, and modeling how a given cell might respond to interventions like gene knockouts or immune signals.
The team observed that performance increases consistently with model size, something already known in general-purpose LLMs. Larger models showed higher BERTScores and better gene overlap in simulated tissue generation.
Authors also explore the use of C2S-Scale for simulating how a cell might respond to a given perturbation—such as a drug treatment, gene knockout, or cytokine exposure. Starting from a baseline “cell sentence” and a textual description of the intervention, the model generates a new sentence representing predicted gene expression changes.
This setup may offer a way to model cellular behavior in silico, potentially helping prioritize experiments or explore hypotheses before committing to wet-lab work. It ties into ongoing discussions around “virtual cells”—computational models that could, in some contexts, act as alternatives to traditional cell lines or animal models.
Models and resources are available on HuggingFace and GitHub.
Who Runs the Tools in AI–Pharma Deals
Citing recent IQVIA data showing over $9.7B in AI/ML pharma deal value for 2024, Converge Bio CEO Dov Gertz points to a structural issue: most tech companies are delivering molecules, not technology platforms. As a result, pharma stays dependent on external outputs, with little ability to run the tools themselves.
According to Gertz, this model limits access to only a few large players who can afford $200M+ deals and leaves no recurring revenue stream for the AI platforms driving the science.
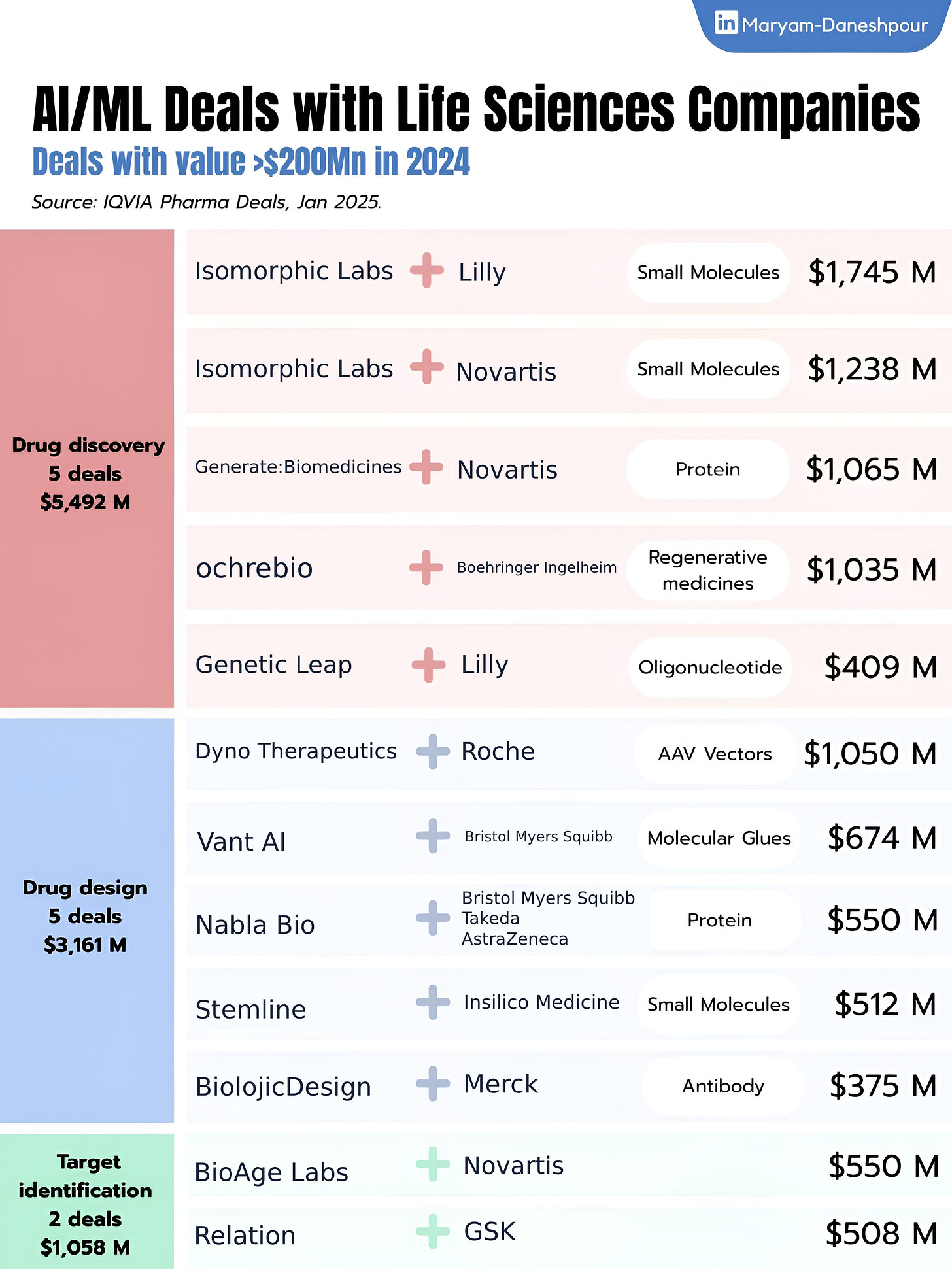
He suggests shifting the model—sell the capability, not just the asset. Instead of one-off licensing deals, let pharma and biotech teams run their own generative AI campaigns, priced per campaign or per use. This would create scalable, recurring value and allow organizations to fine-tune models with proprietary data for their specific use cases.
Four Challenges at the AI-Biology Interface
The Chan Zuckerberg Initiative has outlined four major research efforts focused on combining AI, biology, and engineering to tackle fundamental questions in human health. Each is tied to CZI’s Biohub network and builds on earlier work, including the creation of a one-billion-cell single-cell dataset, real-time inflammation sensors, and AI infrastructure for nonprofit life sciences research.
AI-based virtual cell models
CZI plans to develop models that simulate how cells behave under different conditions using large-scale datasets and machine learning. These models are intended to be openly available and designed for use by researchers to explore cell function, disease progression, and therapeutic response.Next-generation imaging technologies
The Imaging Institute and San Francisco Biohub, led by Scott Fraser, are working on tools to capture biological activity across scales—from proteins to full organisms. The goal is to provide spatial and temporal resolution needed to validate models and understand how cells form tissues and organs.Real-time inflammation sensing in tissues
At the Chicago Biohub, Shana Kelley’s team is creating tools to directly measure inflammation within living tissues. This work aims to capture immune activity in situ, addressing conditions where early detection of inflammatory processes could alter disease outcomes.Immune cell engineering for early detection and treatment
Andrea Califano at the New York Biohub is leading efforts to reprogram immune cells to detect disease signals, report back molecular information, and deliver targeted interventions. The focus is on catching diseases like cancer early and minimizing systemic side effects.
CZI plans to expand its leadership team with a head of AI to oversee the virtual cell challenge, alongside a head of data. The broader goal is to equip researchers with tools that can reveal the underlying mechanisms of disease, supporting prevention and intervention strategies grounded in cell-level understanding.
Read also:
13 Foundation Models: Startups, Industry Updates and the Nobel Prize